AI for Women's Health
Künstliche Intelligenz in der Frauengesundheit
AG Dr. Jacqueline Lammert
Artificial Intelligence for Women's Health
Historically, women have been underrepresented in health research, resulting in insufficient screening and suboptimal treatment strategies. By prioritizing the inclusion of women in AI research and development, we seek to address these disparities and facilitate the advancement of personalized medicine for women.
Our interdisciplinary research group is committed to exploring how AI systems, such as Large Language Models (LLMs), can be further evolved—through close collaboration with clinical experts and patients—into effective decision-support tools for precision medicine. We use AI-driven systems to address the complex information needs of researchers, clinicians, and patients, thereby enhancing the efficacy and precision of medical care.
Research Group Members
- Dr. med. Heike Jansen (Postdoc)
- Maximilian Tschochohei, PhD (Postdoc)
- Florence Heinkelein, BSc (Doctoral candidate in Medicine)
- Leonid Kuligin, MSc (Doctoral candidate in Informatics)
Grants and Awards
- Hightech Young Investigator Award Bavaria 2025 (2025)
- ASCO Journals Editorial Fellowship, JCO Clinical Cancer Informatics (2024-2025)
- Association for Molecular Pathology Europe Young Investigator Award (2024)
- DKTK Travel Grant (2024)
- Google's Gemma Academic Program Award (2024)
- TUM School of Medicine & Health Clinician Scientist Main Program (2024-2025)
- Teaching Award: 'PJ Mentor 2022 for Exceptional Dedication to Teaching at the Faculty of Medicine' (2023)
- DKTK School of Oncology Fellowship (2021-2024)
- TEDxBasel 2019 3 Minute Pop-up Talks Top 3 Finalist (second place) (2019)
- ESMO Merit Award (2019)
- DGHO Travel Grant (2018)
- DGEM Travel Grant (2018)
- DGEM-DGHO Doctoral Scholarship (2018)
- UnternehmerTUM, Prototype your PhD - Winner "Best Pitch" (2018)
- DDG Travel Grant (2018)
- TUM Graduate School Internationalization Grant (2017)
Dr. med. Jacqueline Lammert, B.Sc.
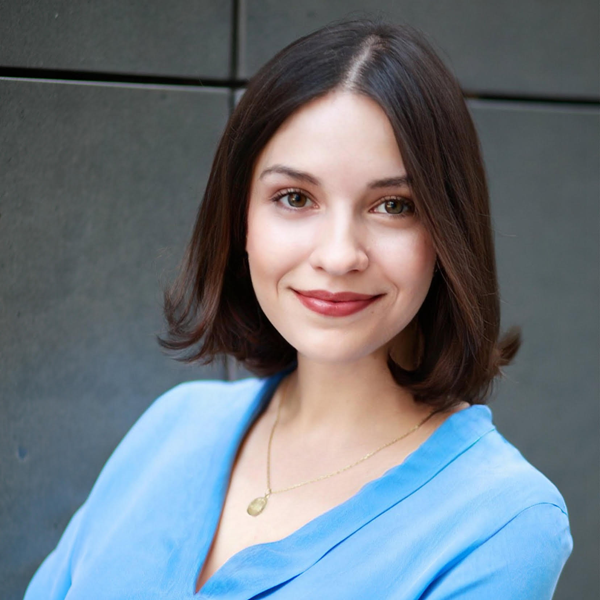
Lead
Clinician Scientist and Spokesperson for Gynecological Precision Oncology at the Center for Personalized Medicine, TUM University Hospital
Maximilian Tschochohei, PhD
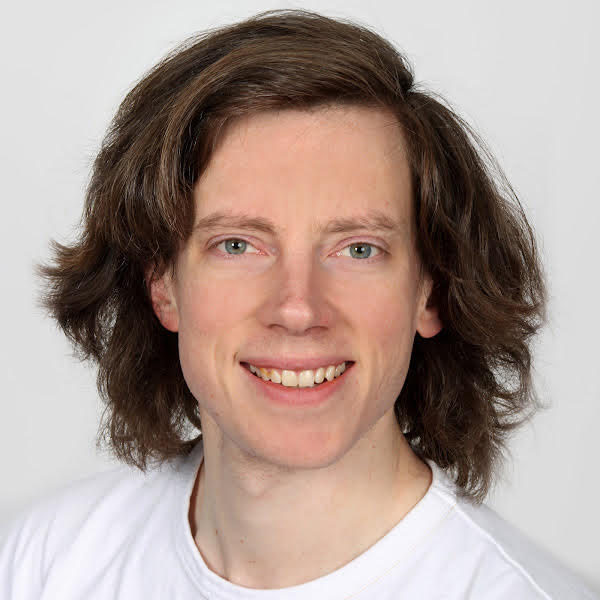
Deputy Lead
Postdoc
Head of AI Engineering
Research Projects
Domain-specific LLM system for decision support in molecular tumour boards (MTBs)
Problem: Precision oncology aims to deliver personalized treatment recommendations based on genomic biomarkers. However, the translation of genomic data into individualized treatment plans is hindered by the fragmentation of medical information across numerous unstructured databases. Delays in diagnostic workflows and the clinical interpretation of biomarker profiles often prevent the timely translation of molecular insights into actionable treatment decisions, see Lancet Oncology Editorial von August 2024(link is external). Previous studies(link is external) have demonstrated that general-purpose large language models (LLMs) are not well-suited for precision oncology therapy recommendations due to their lack of domain specificity.
Our Solution: The MEREDITH (Medical Evidence Retrieval and Data Integration for Tailored Healthcare) LLM system employs Retrieval-Augmented Generation (RAG) to generate therapy recommendations by integrating data from clinical trials, oncological guidelines, therapeutic availability, and trial databases. This system mirrors the approach of experts in Molecular Tumor Boards (MTBs) by interpreting molecular data within a comprehensive medical context.
Status: MEREDITH is capable of assisting precision oncologists in selecting molecularly targeted therapies and identifying suitable clinical trials. Our proof-of-concept study, conducted using fictitious patient data, demonstrated a high level of agreement (94.7%) with human MTB experts.
Details: Publication (JCO Precision Oncology)
Digital Twins in Gynecological Precision Oncology
Problem: Over 50% of all gynecological tumors are classified as rare (incidence of <6 per 100,000 women). The rarity and heterogeneity of these diseases result in a lack of comprehensive clinical guidelines, hindering the quality of care for affected patients. The conventional approach of classifying metastatic tumors by their site of origin often leads to the exclusion of patients with rare tumors from emerging treatment options. Uterine carcinosarcomas, for example, are included in the S3 guidelines for endometrial carcinoma(link is external), with treatment options limited to first-line therapy with carboplatin/paclitaxel. Despite this, median survival has remained poor for decades, typically less than two years. There is an urgent need for novel, clinically evaluated therapeutic strategies.
Our Solution: Through our work, we aim to catalyze a paradigm shift towards individualized, biomarker-driven treatment strategies. This approach facilitates more precise patient stratification, providing the foundation for personalized therapy—particularly in scenarios where large-scale clinical trials are not feasible. To this end, we have developed and piloted a Digital Twin System: utilizing LLM-supported data extraction, structuring, and analysis of both institutional and literature-based data, we have successfully analyzed a cohort of over 400,000 patients and assessed a range of biomarker-based therapeutic options.
Details: Preprint (arXiv)(link is external)
Status: We aim to use the results of our proof-of-concept study to improve treatment outcomes and quality of life for patients with advanced gynecological tumors. This will be implemented as part of a collaborative project beginning in May 2025, with further details to be provided by the end of June 2025.